Week 49: Trusting the Right Tool for the Job
Why measuring HRV accurately starts with the right device
hi there 👋
I hope everything is well.
This blog was prompted by a recent paper showing issues in HRV measurements for the Apple Watch.
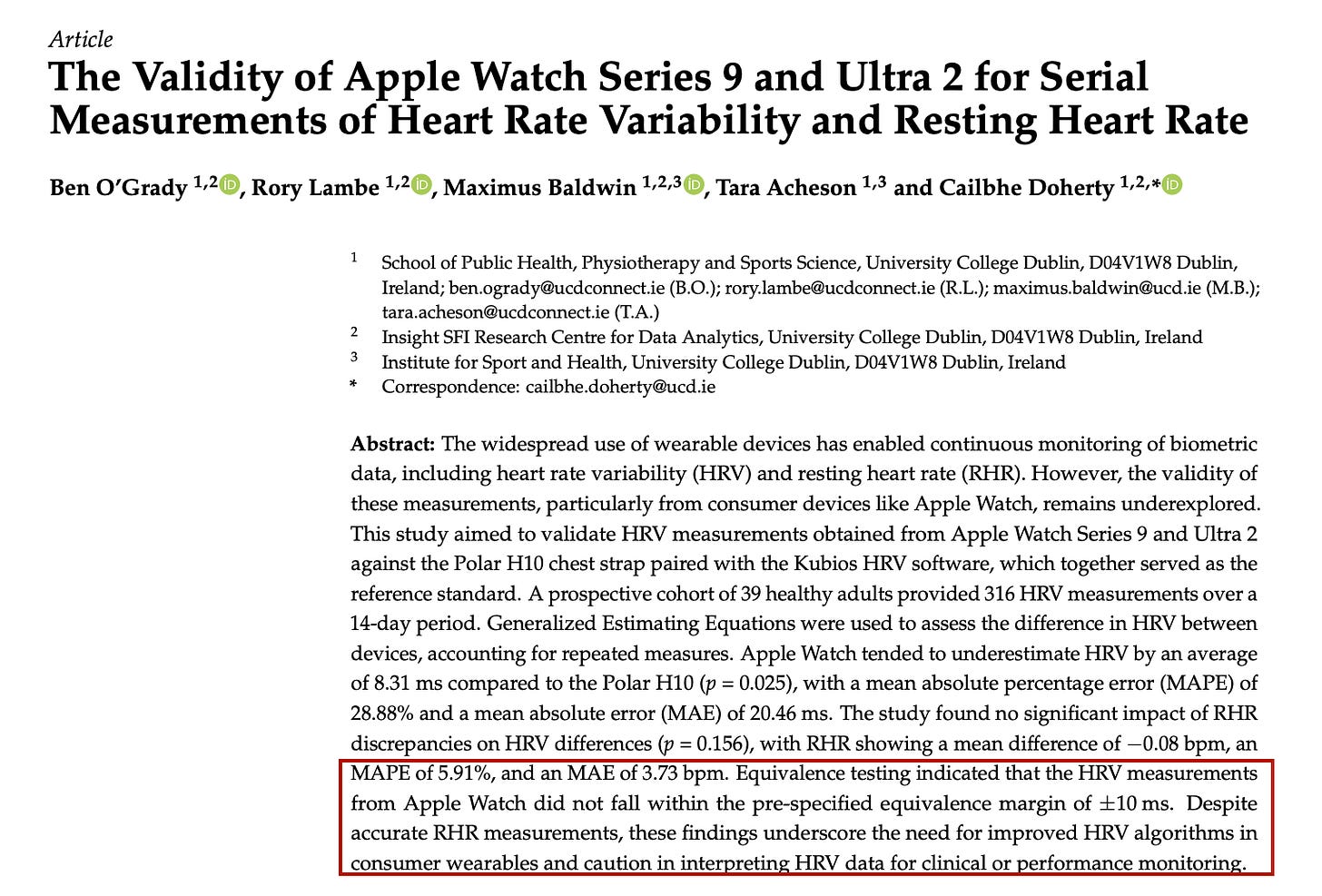
In recent years, we’ve seen an explosion in the popularity of wearable devices that claim to measure everything from sleep to stress, recovery, and more. Among these metrics, heart rate variability (HRV) has emerged as a key parameter for understanding stress and recovery. As readers here will know already, HRV is one of the very few things that wearables can actually measure - even though this is the case only at rest (and we should call it PRV, or pulse rate variability, which can differ from HRV, or the electrical activity of the heart - but that’s another story). All other parameters are either estimated (e.g. sleep time and stages) or completely made up (recovery, readiness, stress, etc.).
Yet, not all HRV measurements are created equal, and recent research highlights the pitfalls of relying on hardware or algorithms not specifically optimized for the job. The prime example of this issue is certainly the Apple Watch (oh dear).
Not only the Apple Watch does not comply with standard protocols to communicate with third-party apps, but Apple Watch's automatically collected data is also subject to changes in a way that makes it unreliable. See for example in the image below the differences in HRV when exporting twice the same data. If your HRV goes from 50ms to 100ms when exporting it a second time, something is really wrong. We cannot trust the processing provided.
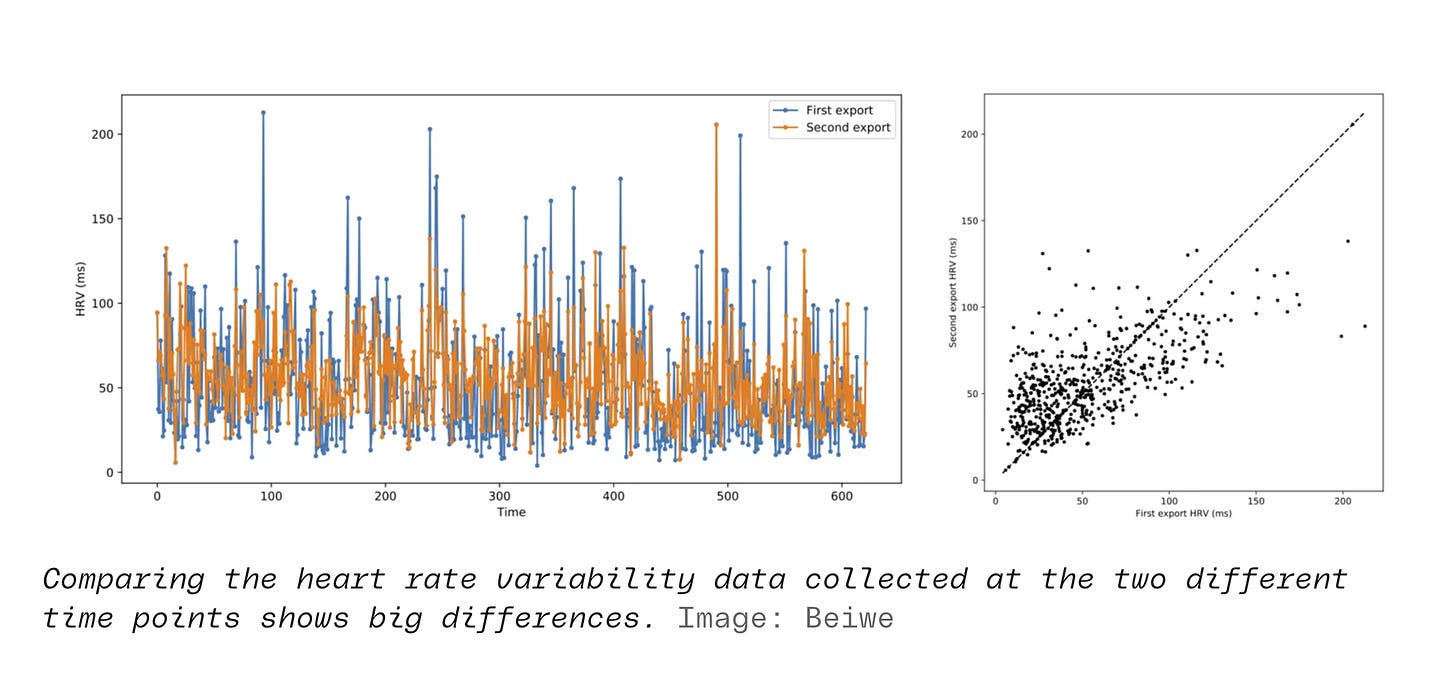
On top of these issues, the recent paper mentioned above shows poor accuracy for the Apple Watch when used to compute HRV, despite good results for heart rate data (at rest). While I’ve used the Apple Watch as an example here, mainly given its popularity and poor performance at the task (no comment on the apps using it as the only data source), this is a much larger problem when using wearables and automatic measurements. For example, years ago, after showing how the only reliable way to use HRV data collected during the night was to average it (learn more here), Whoop quietly changed their algorithms, potentially leading to inconsistencies in users’ data. Similarly, Reddit is full of users whose HRV has changed when upgrading to a new Oura ring.
The first step to avoid the issues above and take control of your measurements and data is to measure intentionally (i.e. first thing in the morning), using a device that either 1) shows you the raw data, e.g. the actual PPG signal, 2) at least provides the RR intervals, or beat to beat data, which can then also be compared with a gold standard (e.g. electrocardiography). HRV is not the easiest signal to measure and is prone to artifacts. Without an accurate and consistent device, we are collecting noise.
The Problem with "All-in-One" Devices
Many wearables today, such as smartwatches and rings, aim to do it all. They track your steps, sleep, workouts, HRV, etc. However, as highlighted in the study linked above about the Apple Watch, the tradeoffs in hardware and algorithm design often result in inconsistent or inaccurate HRV measurements. It is common for users to report quite different HRV values when e.g. upgrading to a newer version of their wearable (or just updating software). Why does this happen?
It boils down to hardware constraints and priorities. A smartwatch for example must be relatively small, lightweight, and have a long battery life. These tradeoffs mean compromises in sensor design, sampling frequency, and artifact correction algorithms. For most of these devices - the Apple Watch in particular - HRV often ends up being an afterthought, not the core functionality of the device. The outcome is then the one we see, with inaccurate data, values changing based on whatever fix is implemented later on, etc.
In contrast, tools designed to do one job, i.e. measuring HRV, like a chest strap or a dedicated HRV app - even using the camera as in HRV4Training - are designed specifically for this purpose and this purpose only. These tools are optimized for accurate data collection over a short period without being burdened by competing priorities and will provide you with more accurate data.
The same considerations apply to software aggregators - you can get all data in one place, but none of the meaning. In contrast, using different tools, carefully designed with the specific goal of helping you interpret one type of data, can provide more value.
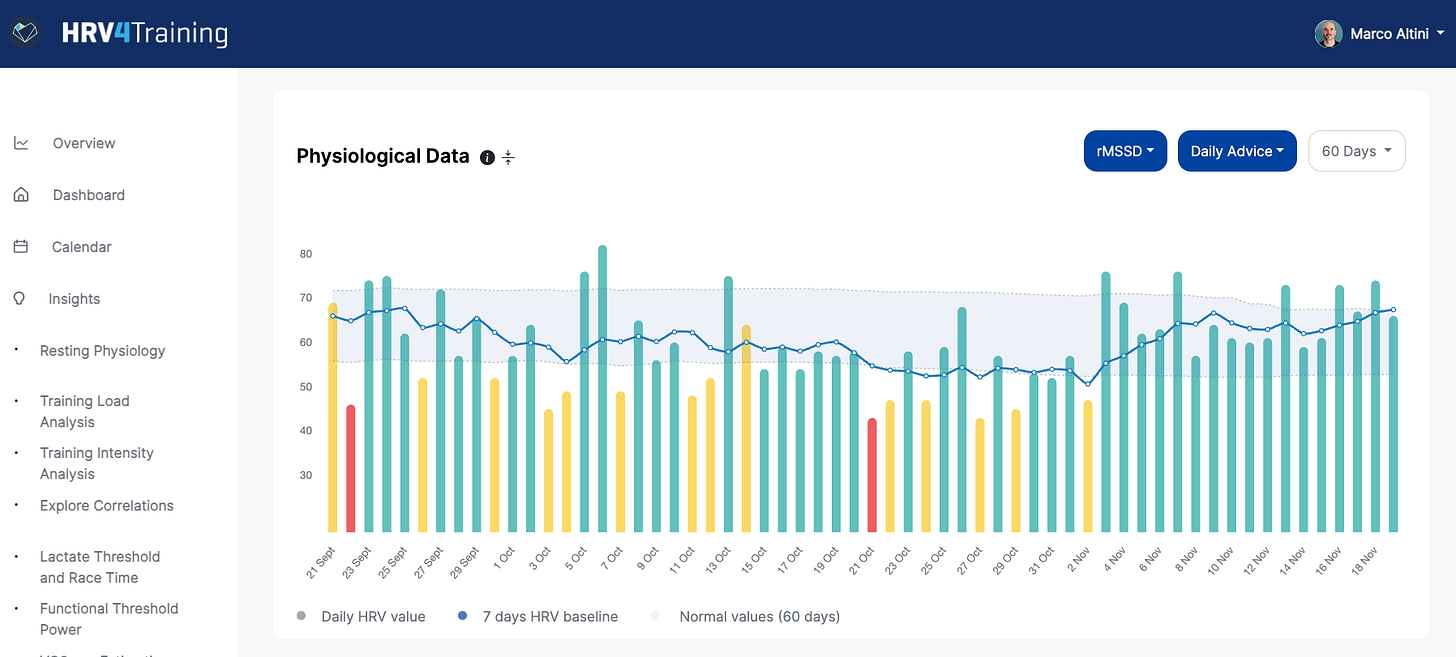
Personally, I use a chest strap (Polar H10 paired with the HRV Logger app) every time I want to:
Check HRV before and after exercise to assess exercise intensity (learn more here).
Do a deep breathing exercise using the HRV4Biofeedback app.
Check ectopic beats to keep track of premature ventricular contractions or else (also with the HRV Logger).
On the other hand, I use the phone camera in HRV4Training to assess my morning physiology.
I spent the last 11 years of my life building this app with the one goal of measuring HRV accurately, and as such, I trust it (and I have validated it against electrocardiography). If you do not trust this method, just use a chest strap for your morning measurements with HRV4Training as well. You will be one of the few people that actually knows their HRV, and not just their PRV.
Wrap up
HRV is a sensitive metric. Small changes in data collection methodology—sampling frequency, sensor placement, or artifact correction algorithms—can lead to significantly different outcomes. For instance, when wearable manufacturers update their algorithms or hardware, your HRV trend might suddenly shift, not because your physiology has changed, but because the device’s interpretation of your data has.
It’s tempting to rely on a single wearable or platform to track everything. The convenience of having all your data in one place is hard to resist. But the reality is that this convenience often comes at the expense of accuracy. When HRV is just one of many features, it’s unlikely to receive the dedicated attention required to ensure high-quality measurements.
Imagine using HRV to guide your recovery, only to find out that your "improvement" was actually due to a software update or a change in the device's artifact correction method: this is a lot more common than you’d think.
Using a wearable - even an accurate one - and automated data collection, you never have control over firmware or software updates that might change your data in ways that mess up your analysis and interpretation.
If you care about measuring your HRV accurately, don’t settle for a general-purpose device where HRV is an afterthought. Instead, consider using tools designed specifically for HRV. Whether it’s a chest strap or a camera-based app, these tools provide the reliability needed to take control, measure intentionally and make meaningful decisions based on your data.
For those seeking the most accurate HRV measurements, a chest strap remains the gold standard. Chest straps are specifically designed to capture precise beat-to-beat heart rate data (RR intervals), with high sampling rates and minimal noise. These devices don’t have to worry about conserving battery life for days or fitting a dozen sensors into a sleek form factor, or powering a screen.
They do one job—and they do it exceptionally well.
Remember, it’s not all about convenience; it’s about trust. Trust the right tool for the job, and you’ll be on the path to meaningful insights and better health and performance.
November articles and updates
Below is my personal newsletter including articles and updates from November 2024. A part from Apple Watch’s failed validation, I cover HRV normalization, sleep time detection issues, and more.
Thank you for reading.
Discount for Pro: 20% off 🖥️
HRV4Training Pro is the ultimate platform to help you analyze and interpret your physiological data, for individuals and teams.
You can find a guide here.
Try HRV4Training Pro for free at HRVTraining.web.app or use promo code SCIENCE for 20% off.
In the app, Pro brings the normal values view, which can help contextualizing longer-term changes, as well as rMSSD on the homepage, see an example below:
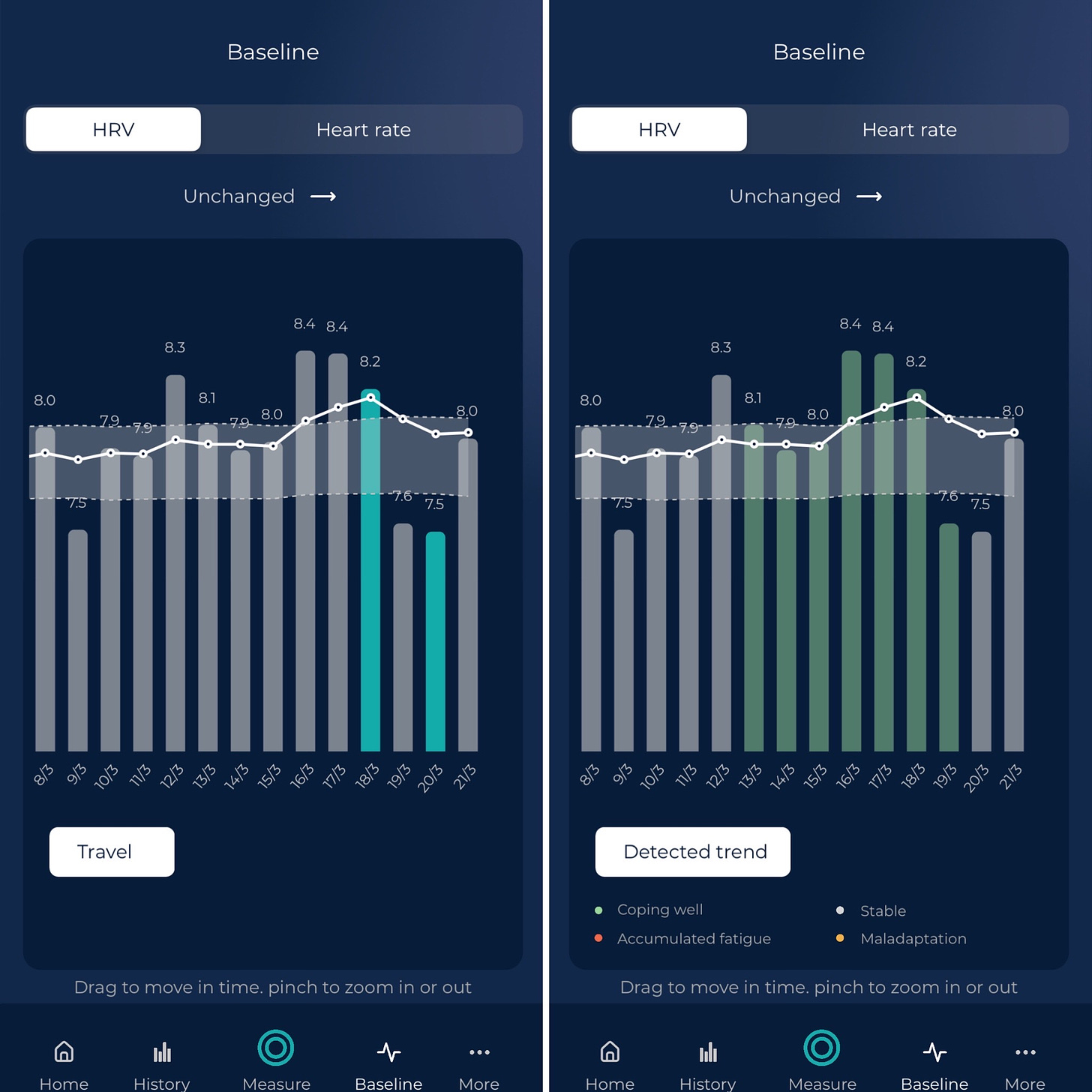
See you next week!
Marco holds a PhD cum laude in applied machine learning, a M.Sc. cum laude in computer science engineering, and a M.Sc. cum laude in human movement sciences and high-performance coaching.
He has published more than 50 papers and patents at the intersection between physiology, health, technology, and human performance.
He is co-founder of HRV4Training, advisor at Oura, guest lecturer at VU Amsterdam, and editor for IEEE Pervasive Computing Magazine. He loves running.
Social:
Hi, I’m a HRV4TPro subscriber. Recently updated to iPhone 18 and anecdotally seem to be getting a lot more sub-optimal readings for HRV. Is there anything about this new device/camera that impacts capturing HRV?
Dear Marco,
Thanks for your excellent comments. I was a bit confused though reading the last one about ‘silent upgrades’ of algoritmes: “Reddit is full of users whose HRV has changed when upgrading to a new Oura ring”. I’m fond of my Oura ring and always trusted the results but recently puzzled about the strange outcomes from very low to surprisingly high. Could you comment on this more being a consultant of Oura.