Week 41: HRV in team sports
hi there 👋
I hope everything is well.
Today I’d like to cover a recent study looking at HRV in team sports.
In particular, Fergus K. O’Connor and co-authors have used HRV4Training for a few years in team settings and reported part of their findings in their latest article, titled “A two-year examination of the relation between internal and external load and HRV in Australian Rules Football“, which you can find here.
While HRV is widely used in individual sports, fewer studies are looking at measures of resting physiology in team settings. Here, I briefly cover the protocol used and the main findings of Fergus’ work.
Protocol
The protocol for using the HRV4Training app in this study was as follows:
Data Collection: Athletes used the HRV4Training app to record their HRV upon waking each morning (this is the protocol we recommend, especially for athletes, see here). The recordings were taken using a smartphone camera placed over the fingertip, which captured the photoplethysmographic (PPG) data. The app then calculated rMSSD (root mean square of successive differences between heartbeats), which is a standard HRV metric representing parasympathetic activity.
Measurement Validity: The HRV data was included in the analysis only if it met the app's criteria for an "optimal" measurement, which required less than 6% artifacts in the recording. The HRV4Training app automatically identified and discarded faulty readings. Note that the vast majority of tools out there (e.g. pretty much all wearables) pretend to provide high-quality data all the time, making it impossible for users and researchers to determine if the data was indeed of high quality, or if it was artifacted due to movement, arrhythmias, and all sorts of other issues that have a large impact on HRV data. If your tool of choice does not report signal quality for the recorded data, change tool.
Time Frame: HRV data was recorded over a period that included two pre-season and two in-season periods, providing a comprehensive view of the athletes' recovery and stress responses across different training intensities and phases. This is interesting as different responses have been reported in literature during pre-seasion, when athletes get back into structured training (see also my previous blog, here).
HRV4Training provided a practical and non-invasive method of monitoring stress and recovery in a team-sport environment. Its ease of use made it suitable for daily monitoring across large groups of athletes, and its reliability in reflecting autonomic activity was validated through comparison with gold-standard methods like ECG (validation here).
Findings
The authors looked at the relationship between sprinting, high-speed running (i.e. running at moderate velocities, without sprinting), and HRV. The relationships were analyzed in the pre-season and regular season, as well as divided based on athletes’ experience.
Key findings:
Training Load and HRV: increased sprint distance over 3 days led to decreased HRV, particularly during the pre-season. In contrast, high-speed running was associated with increased HRV. From this, we can derive that when fitness is lower, such as in the pre-season, sprinting, with its high intensity and higher physiological demands, makes it harder for athletes to bounce back quickly from training stress, as shown by a suppressed HRV. During the season we have that a larger amount of higher speed running (but not sprinting) was associated with higher HRV. In my view, this points in a similar direction, as moderate-intensity, sustained running might be less taxing on the nervous system and could contribute to fitness adaptations rather than fatigue.
Experience Matters: players with fewer years of experience showed more pronounced decreases in HRV with higher cumulative load compared to more experienced athletes. As athletes go over multiple seasons, they might become better at managing the load, as reflected in a better HRV profile.
Takeaways
What do we make of this study and its results in terms of practical applicability?
When we include HRV as a monitoring tool, we aim to modulate training intensity based on individual athlete responses. By monitoring HRV with tools like HRV4Training, athletes, and coaches can gain insights into how well an athlete is recovering from various types of training. A drop in HRV may signal a need for increased recovery or reduced intensity in upcoming sessions, while stable or increasing HRV might indicate readiness for more intense training or simply reflect the desired adaptation to the current training phase.
Sprinting, while essential for performance, can heavily tax the autonomic system, especially during high-load periods or when athletes are not as fit yet, such as during the pre-season. Athletes could balance sprint training with recovery or lower-intensity running to avoid overloading the nervous system or could try prioritizing recovery (e.g. sleep and nutrition) to see if a better response is captured.
Finally, younger or less experienced athletes might require a more gradual increase in sprint-based activities to build resilience, whereas more experienced athletes may be able to handle higher sprint volumes without the same level of autonomic fatigue, according to the data reported in this study.
This personalized approach to training load management, facilitated by HRV monitoring, should lead to optimized training adaptations while minimizing risks.
If you are interested in using HRV with your team, you can reach us here.
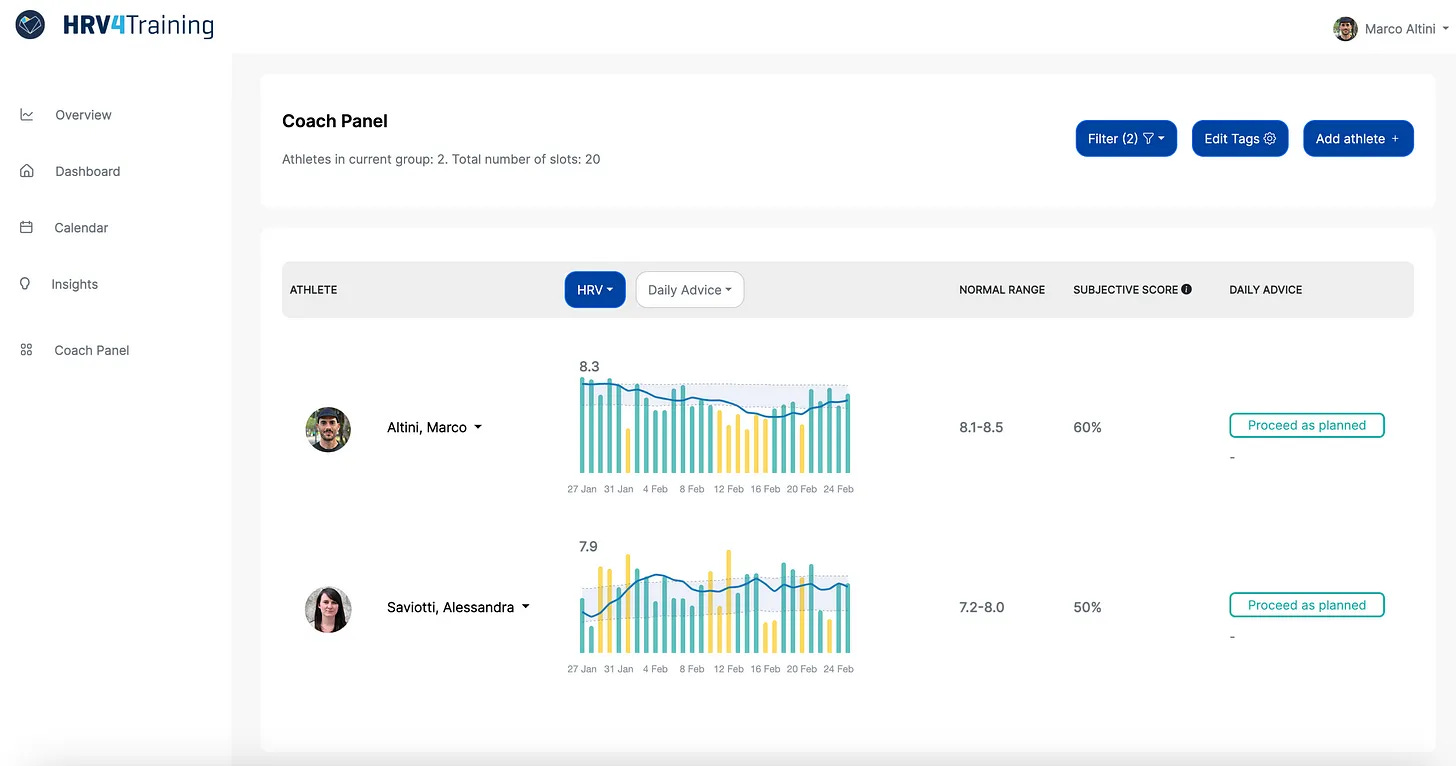
Ambassador Program 2025
Applications to become HRV4Training ambassadors for next year are still open. You can learn more and apply, here.
Discount for Pro: 20% off 🖥️
HRV4Training Pro is the ultimate platform to help you analyze and interpret your physiological data, for individuals and teams.
You can find a guide here.
Try HRV4Training Pro for free at HRVTraining.web.app or use promo code SCIENCE for 20% off.
In the app, Pro brings the normal values view, which can help contextualizing longer-term changes, as well as rMSSD on the homepage, see an example below:
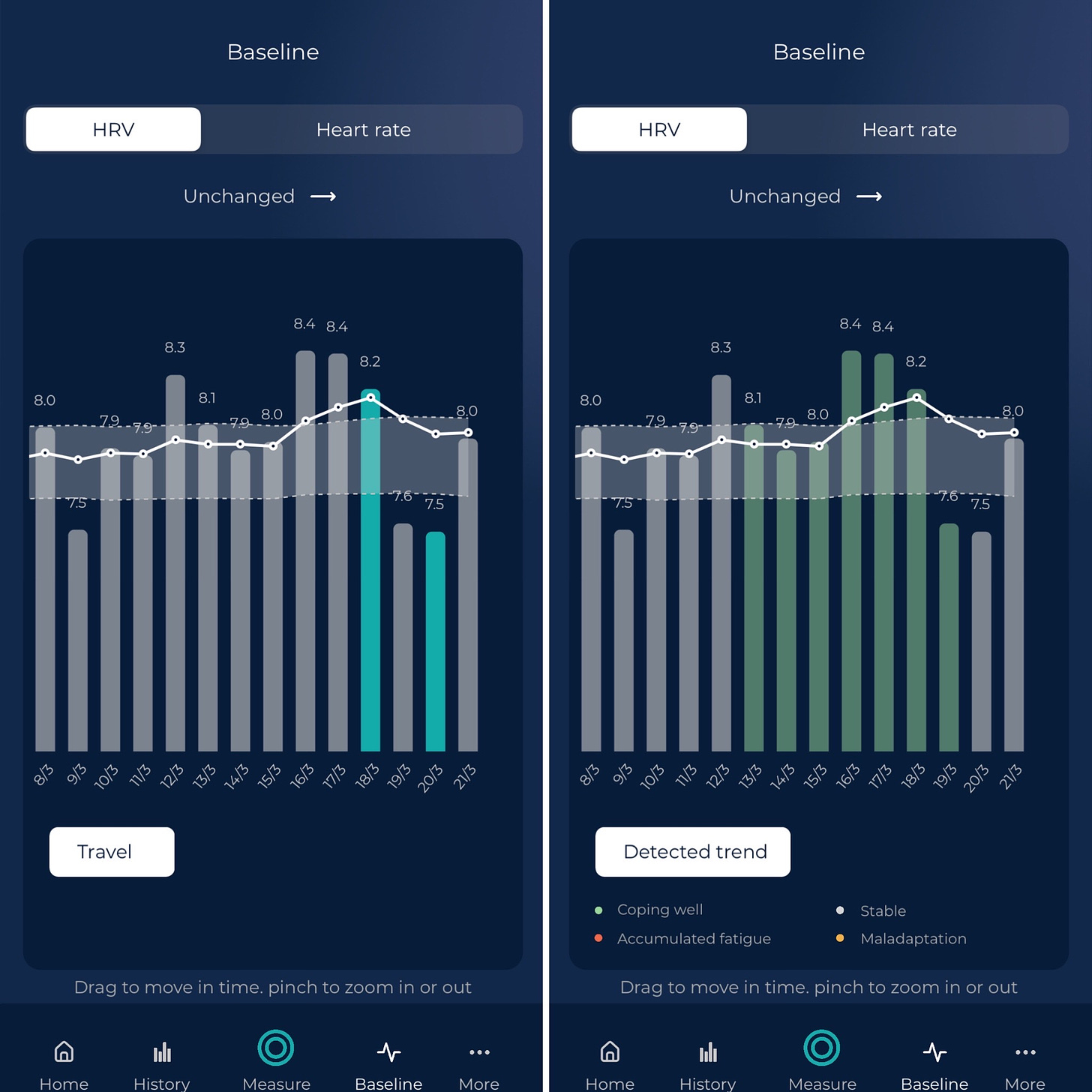
See you next week!
Marco holds a PhD cum laude in applied machine learning, a M.Sc. cum laude in computer science engineering, and a M.Sc. cum laude in human movement sciences and high-performance coaching.
He has published more than 50 papers and patents at the intersection between physiology, health, technology, and human performance.
He is co-founder of HRV4Training, advisor at Oura, guest lecturer at VU Amsterdam, and editor for IEEE Pervasive Computing Magazine. He loves running.
Social: